Translate this page into:
Artificial Intelligence in Cardiac Critical Care: Current Insights and Future Prospects
*Corresponding author: Devishree Das, Department of Anaesthesiology and Critical Care, All India Institute of Medical Sciences, Bhubaneswar, Odisha, India. devishreedas111@gmail.com
-
Received: ,
Accepted: ,
How to cite this article: Das D, Choudhury M. Artificial Intelligence in Cardiac Critical Care: Current Insights and Future Prospects. J Card Crit Care TSS. 2024;8:189-94. doi: 10.25259/JCCC_67_2023
Abstract
Cardiac critical care (CCC) involves a heterogenous group of critically ill patients and poses an ever-growing challenge to the healthcare system. Moreover, their clinical outcome improved to an unprecedented level due to significant improvements in the critical care practice. Artificial intelligence (AI) is an emerging transdisciplinary field that involves multidomain and multidimensional computerized data to handle heterogeneity, complexity, and acuity which were the major limitations of conventional critical care practice. AI employs machine learning techniques for disease identification from an exhaustive list of differential diagnoses, prediction of disease evolution and its diverse manifestations, dynamic risk calculation, optimal sequential decision-making solutions, and trajected prediction of clinical deterioration or recovery. This review highlights the current advances and implementations of AI algorithms in CCC practice with respect to sepsis, heart failure, arrhythmia, and various cardiovascular diseases.
Keywords
Artificial intelligence
Cardiac critical care
Cardiovascular disease
Critical care medicine
INTRODUCTION
Cardiac critical care (CCC) encompasses a major burden globally, accompanied by increasing mortality. It involves a wide variety of cardiovascular diseases with their diverse outcome. Therefore, artificial intelligence (AI) helps in the assimilation of disease complexity, handling heterogeneity of clinical presentation, risk stratification, anticipating clinical worsening, and proficiency in providing early treatment strategies in a better way and an adjunct to conventional critical care practice.
AI is a rapidly developing technology that amalgamates material science, neuronal networks, and computer science to develop algorithms that facilitate the complex network of action.[1] AI aims to simulate the human brain in early recognition and optimal decision-making. With exponential advancement in technologies, AI has become an inevitable part of critical care medicine, cardiovascular illness, and CCC.[2]
This review emphasizes the current supremacy, application, and pitfall of AI in CCC and also enlightens the anticipated challenges and future prospects.
HISTORICAL PERSPECTIVE OF AI
In the early 1950s, Alan Turing investigated the possible mathematical algorithm attributed to building intelligent machines; however, these machines were devoid of functional capabilities to implement the theoretical knowledge of AI.[3] In 1955, McCarthy coined the term AI.[3] With the evolution of computer power, clinical decision-making emerged in the 1990s. The initial application of AI in cardiac setup was the evolution of self-learning neuronal networks on electrocardiography (ECG).[4] Over time, AI progressed from computer games to precise disease identification by merging various multi-faceted imaging, AI-aided monitoring techniques, and precise treatment strategies.[5]
AI TECHNIQUE
AI techniques are based on machine learning (ML) that includes various complex mathematical algorithms between input and output by presuming multiple data sets to predict new data sets. ML can be supervised, unsupervised, semi-supervised, or reinforcement learning [Figure 1]. However, deep learning (DL) involves minimal data arbitration [Figure 2]. Convolutional neural network (CNN) involves the DL algorithm, which is based on the visual cortex of the human brain. It is specifically designed for image processing and implementation.
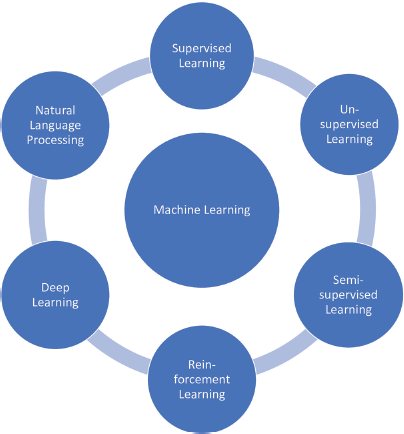
- Artificial intelligence techniques.
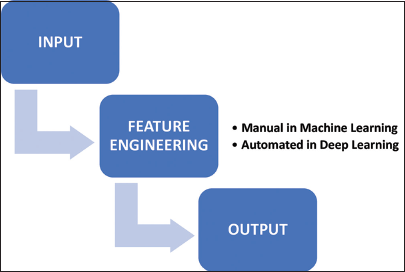
- Comparing machine learning and deep learning approaches.
AI is categorized into predictive AI and actionable AI. In a predictive model, AI offers early warning of possible adverse events so that the clinician can pre-emptively initiate appropriate intervention. Whereas actionable AI performs interference tasks and could even advise treatment options for the best predicted outcome [Figure 3].
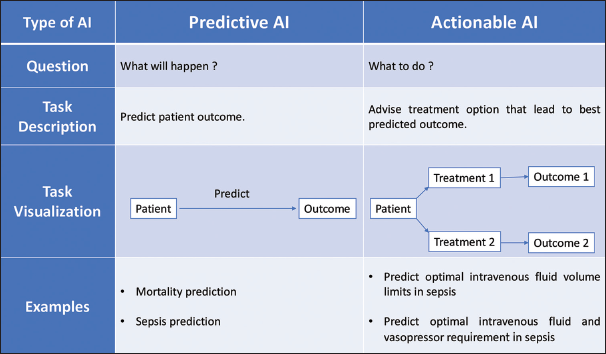
- Artificial intelligence types in critical care practice.
APPLICATIONS OF AI IN CCC
Disease identification
Identifying the criticality of a disease from an extensive list of differential diagnoses can become easier with the help of AI. For example, pulmonary edema secondary to heart failure can be discriminated from pulmonary pathology using an ML model[6], and moreover, the amount of edema in the lungs can also be quantified using a semi-supervised variational autoencoder.[7] Similarly, during the COVID-19 pandemic, severe acute respiratory syndrome (SARS) was detected by processing imaging data from patients using an AI model.[8]
Disease evolution and risk prediction
In the critical care unit, expeditious clinical deterioration is not uncommon and may be irreversible and life-threatening if diagnosed late. Thus, early prediction and stratification of hemodynamic decompensation are necessary for timely action. Tachycardia is an early sign of observed disparity from the normal range before shock, and it can be predicted 75 min before shock using a random forest model-based normalized dynamic risk score trajectory.[9]
AI-driven models can forecast the evolution of SARS due to COVID-19 by utilizing various clinical parameters and imaging modalities.[10] Even cardiopulmonary arrest can be predicted with an electronic Cardiac Arrest Risk Triage score, which was shown to be non-inferior as compared to traditional scoring systems.[11] Even the Weibull-Cox proportional hazards model, on the basis of multifarious clinical databases and collective vital parameters, can predict sepsis.[12]
Disease heterogeneity and decision-making
Cardiovascular criticality is complex and rarely has typical manifestation. Rather it has inherent heterogeneity, which afterward can complicate the underlying disease course and may incite multiorgan dysfunction. Furthermore, the composite critical state transits so rapidly that clinicians cannot make decisions on the basis of recent investigations. AI could delineate heterogeneity and criticality and, therefore, trigger individualized management in accordance with current guidelines.
A parsimonious model was developed and validated for latent stage analysis of acute respiratory distress syndrome (ARDS) using various classifier variables (interleukin-6, 8, tumor necrosis factor receptor, protein C, bicarbonate levels, and vasopressors requirement).[13] This ML-based ARDS classification has extended and integrated the knowledge for assessing and managing such complex situations. Furthermore, for patients on ventilatory support, timed data with almost 44 characteristics were retrieved with the help of the reinforcement learning model (Markov Decision Process) to provide better patient outcomes as compared to clinician conventional care with regard to 90-day and intensive care unit (ICU) mortality.[14] AI helps in each step of diagnosis and treatment of sepsis, such as early detection by predictive analytics, AI-driven real-time monitoring, risk stratification, personalized treatment plans, antibiotics stewardship, fluid responsiveness, outcome prediction, and integrated electronic health records. Applying reinforcement learning-based algorithms for sepsis management, AI can provide timely optimal decision-making and generate appropriate individualized solutions.[15]
IMPLICATION OF AI IN CARDIOVASCULAR DISEASE
Coronary artery disease (CAD)
Acute coronary syndrome (ACS) is the most common type of cardiac illness and the major cause of mortality nowadays. ML-based algorithms play a pivotal role in the prompt diagnosis, risk stratification, disease prognostication, and treatment protocol for ACS. Recently, a CNN algorithm using 40 variables such as ECG, biomarkers, and history was validated to predict the requirement of angiography in ACS presenting to the emergency department.[16] Similarly, another CNN developed from 42 demographic and clinical variables that predict non-ST elevation myocardial infarct.[17] AI algorithms using the support vector machine (SVM) model can also derive short-term as well as long-term in hospital mortality in patients with ACS.[18] A random forest ML-based technique was introduced to measure coronary arterial calcium, which can be derived automatically from computed tomography (CT) imaging. This algorithm has a better correlation coefficient and importantly, shortened the processing times in comparison to manual interpretation.[19] Another novel study used a boosted ensemble ML algorithm (LogitBoost)-based technique to evaluate myocardial perfusion single-photon emission CT scan of suspected and low likelihood of CAD for risk stratification.[20]
Valvular heart disease
Valvular pathologies are well diagnosed by their characteristic murmur, and therefore, AI-based algorithms were incorporated into phonocardiograms for clinical decision-making. SVM classifier was developed to classify abnormal heart sounds, which is an AI technology.[21] AI algorithms can even identify minimal discrimination and pattern in pixel density and thereby, widely applied to echocardiography. An ML algorithm was introduced in patients with aortic stenosis undergoing transcatheter aortic valve replacement (TAVR) to measure the aortic valve annulus. Furthermore, in hospital mortality after TAVR can be predicted using artificial neural network (ANN), logistic regression technique, and random forest ML algorithms.[22] Similarly, another ML-based algorithm was developed for measuring the mitral annular dimensions, and it is significantly less time consuming.[23]
Electrophysiology
Arrhythmias are common with underlying cardiac pathology, especially atrial fibrillation, which is the most prevalent arrhythmia. Nowadays, AI adds new insights for diagnosis and risk stratification to the key diagnostic tool ECG. Several recent trials incorporate ANN on single, 3, and 12 lead ECGs to accurately distinguish and diagnose arrhythmias. Hannun et al. and Raghunath et al. used deep neural networks (DNN) for the classification of ECG into rhythm classes and to predict all-cause mortality.[24,25] ML algorithms can be used to detect electrolyte derangements and predict the future incidence of arrhythmias.
Cardiac failure
Cardiac failure is a complex entity where cardiac output does not meet metabolic demand. AI-based predictive modeling and automated cardiac function measurements reinforce diagnostic capacity and decision-making as compared to conventional echocardiography. Moreover, advanced AI like “HeartModel A.I.” uses AI segmentation and 3D echocardiographic algorithm to quantify cardiac volumes automatically and it was validated by Medvedofsky et al.[26] Son et al. and Kang et al., developed various AI-based algorithms, SVM-based models, and ANN in patients with HF for risk assessment, medication adherence, predicting morbidity and mortality, and repeated hospitalizations.[27,28] The LINK-HF study and Akbilgic et al., developed a CNN-based ECG-AI model were examples of the advancement of AI technology in the management of heart failure.[29,30]
FUTURE PROSPECTS AND CHALLENGES
Future AI models can be planned to maximize patient welfare and minimize risks to the patients with proper utilization of limited resources. The Randomized Embedded Multifactorial Additive Platform for Community-Acquired Pneumonia is one such example. It is adopted from the Bayesian inference model, and this multicentric trial allows randomization with multiple causal inferences, creates robust intervention arms with multiple patient subgroups, provides response-dependent randomization, and provides a new perpetual enrollment platform along the evaluation of the initial treatments. This platform not only identifies optimal management strategies for community-acquired pneumonia but is also used during the COVID-19 pandemic for better treatment and survival of critically ill patients.[31]
AI-enabled devices are costlier to set up; however, carrying out repetitive, simple tasks more accurately and substantially faster without medical errors makes it cost-effective. However, low-cost wearable devices, smart monitoring systems, point-of-care diagnostics and imaging devices, tensor flow-based predictive outcomes, Tele ICU, etc., are more affordable AI-enabled systems and can be integrated into existing hospital infrastructure. Moreover, the incorporation of AI needs additional training such as operational training, technical training, and troubleshooting for adaptation to new tools to aid clinical decision-making.
Real-time AI models with expeditious data pre-processing techniques can be updated rapidly with new inputs so that they can be used in the real scenario. The output should also be quickly delivered to the patients. Once the AI models achieve such tremendous performance and are ready to helpful in a real-life scenario, stringent efforts should be carried out for appropriate implementation strategies as well as quality assessment.[32]
CONCLUSION
The global burden of cardiac illness and its criticality requires timely diagnosis, effective treatment, and stringent follow-up for better patient outcomes and appropriate utilization of healthcare resources. Fortunately, AI algorithms are becoming a powerful tool in drawing pertinent clinical inputs derived from numerous investigation reports, monitoring tools, and diagnostic datasets and utilizing these data for building ML, ANN, DNN, and SVM-based models and helping disease identification, risk stratification, and clinical decision-making. However, necessary efforts should be taken to ensure minimum bias using multicenter, heterogeneous patients for enhancement of the AI solution and their assimilation with the healthcare system.
Ethical approval
Institutional Review Board approval is not required.
Declaration of patient consent
Patient’s consent not required as there are no patients in this study.
Conflicts of interest
Dr. Minati Choudhury is on the editorial board of the Journal.
Use of artificial intelligence (AI)-assisted technology for manuscript preparation
The authors confirm that there was no use of artificial intelligence (AI)-assisted technology for assisting in the writing or editing of the manuscript, and no images were manipulated using AI.
Financial support and sponsorship
Nil.
References
- Artificial Intelligence in Personalized Cardiovascular Medicine and Cardiovascular Imaging. Cardiovasc Diagn Ther. 2021;11:911-23.
- [CrossRef] [Google Scholar]
- Predicting Adverse Hemodynamic Events in Critically Ill Patients. Curr Opin Crit Care. 2018;24:196-203.
- [CrossRef] [Google Scholar]
- History of Artificial Intelligence in Medicine. Gastrointest Endosc. 2020;92:807-12.
- [CrossRef] [Google Scholar]
- Self-Learning Neural Networks in Electrocardiography. J Electrocardiol. 1990;23:200-2.
- [CrossRef] [Google Scholar]
- Artificial Intelligence (AI) and Cardiovascular Diseases: An Unexpected Alliance. Cardiol Res Pract. 2020;2020:4972346.
- [CrossRef] [Google Scholar]
- Chest Radiographs in Congestive Heart Failure: Visualizing Neural Network Learning. Radiology. 2019;290:514-22.
- [CrossRef] [Google Scholar]
- Deep Learning to Quantify Pulmonary Edema in Chest Radiographs. Radiol Artif Intell. 2021;3:e190228.
- [CrossRef] [Google Scholar]
- Using Artificial Intelligence to Detect COVID-19 and Community-acquired Pneumonia Based on Pulmonary CT: Evaluation of the Diagnostic Accuracy. Radiology. 2020;296:E65-71.
- [CrossRef] [Google Scholar]
- Predicting Tachycardia as a Surrogate for Instability in the Intensive Care Unit. J Clin Monit Comput. 2019;33:973-85.
- [CrossRef] [Google Scholar]
- Integrating Deep Learning CT-scan Model, Biological and Clinical Variables to Predict Severity of COVID-19 Patients. Nat Commun. 2021;12:634.
- [CrossRef] [Google Scholar]
- Validating the Electronic Cardiac Arrest Risk Triage (eCART) Score for Risk Stratification of Surgical Inpatients in the Postoperative Setting: Retrospective Cohort Study. Ann Surg. 2019;269:1059-63.
- [CrossRef] [Google Scholar]
- An Interpretable Machine Learning Model for Accurate Prediction of Sepsis in the ICU. Crit Care Med. 2018;46:547-53.
- [CrossRef] [Google Scholar]
- Development and Validation of Parsimonious Algorithms to Classify Acute Respiratory Distress Syndrome Phenotypes: A Secondary Analysis of Randomised Controlled Trials. Lancet Respir Med. 2020;8:247-57.
- [CrossRef] [Google Scholar]
- Development and Validation of a Reinforcement Learning Algorithm to Dynamically Optimize Mechanical Ventilation in Critical Care. NPJ Digit Med. 2021;4:32.
- [CrossRef] [Google Scholar]
- The Artificial Intelligence Clinician Learns Optimal Treatment Strategies for Sepsis in Intensive Care. Nat Med. 2018;24:1716-20.
- [CrossRef] [Google Scholar]
- A Neural Network Aid for the Early Diagnosis of Cardiac Ischemia in Patients Presenting to the Emergency Department with Chest Pain. Ann Emerg Med. 2002;40:575-83.
- [CrossRef] [Google Scholar]
- An Artificial Intelligence Approach to Early Predict NonST-elevation Myocardial Infarction Patients with Chest Pain. Comput Methods Programs Biomed. 2019;173:109-17.
- [CrossRef] [Google Scholar]
- Extensive Phenotype Data and Machine Learning in Prediction of Mortality in Acute Coronary Syndrome-The MADDEC Study. Ann Med. 2019;51:156-63.
- [CrossRef] [Google Scholar]
- Automatic Coronary Calcium Scoring in Non-Contrast-Enhanced ECG-Triggered Cardiac CT With Ambiguity Detection. IEEE Trans Med Imaging. 2015;34:1867-78.
- [CrossRef] [Google Scholar]
- Prediction of Revascularization after Myocardial Perfusion SPECT by Machine Learning in a Large Population. J Nucl Cardiol. 2015;22:877-84.
- [CrossRef] [Google Scholar]
- Artificial Intelligence-Assisted Auscultation of Heart Murmurs: Validation by Virtual Clinical Trial. Pediatr Cardiol. 2019;40:623-9.
- [CrossRef] [Google Scholar]
- 3D Echocardiographic Analysis of Aortic Annulus for Transcatheter Aortic Valve Replacement Using Novel Aortic Valve Quantification Software: Comparison with Computed Tomography. Echocardiography. 2017;34:690-9.
- [CrossRef] [Google Scholar]
- Efficacy and Accuracy of Novel Automated Mitral Valve Quantification: Three-Dimensional Transesophageal Echocardiographic Study. Echocardiography. 2016;33:756-63.
- [CrossRef] [Google Scholar]
- Cardiologist-level Arrhythmia Detection and Classification in Ambulatory Electrocardiograms Using a Deep Neural Network. Nat Med. 2019;25:65-9.
- [CrossRef] [Google Scholar]
- Prediction of Mortality from 12-lead Electrocardiogram Voltage Data Using a Deep Neural Network. Nat Med. 2020;26:886-91.
- [CrossRef] [Google Scholar]
- Three-dimensional Echocardiographic Quantification of the Left-heart Chambers Using an Automated Adaptive Analytics Algorithm: Multicentre Validation Study. Eur Heart J Cardiovasc Imaging. 2018;19:47-58.
- [CrossRef] [Google Scholar]
- Application of Support Vector Machine for Prediction of Medication Adherence in Heart Failure Patients. Healthc Inform Res. 2010;16:253-9.
- [CrossRef] [Google Scholar]
- Utilizing Home Healthcare Electronic Health Records for Telehomecare Patients with Heart Failure: A Decision Tree Approach to Detect Associations With Rehospitalizations. Comput Inform Nurs. 2016;34:175-82.
- [CrossRef] [Google Scholar]
- Continuous Wearable Monitoring Analytics Predict Heart Failure Hospitalization: The LINK-HF Multicenter Study. Circ Heart Fail. 2020;13:e006513.
- [CrossRef] [Google Scholar]
- ECG-AI: Electrocardiographic Artificial Intelligence Model for Prediction of Heart Failure. Eur Heart J Digit Health. 2021;2:626-34.
- [CrossRef] [Google Scholar]
- The REMAP-CAP (Randomized Embedded Multifactorial Adaptive Platform for Community-acquired Pneumonia) Study, Rationale and Design. Ann Am Thorac Soc. 2020;17:879-91.
- [CrossRef] [Google Scholar]
- Artificial Intelligence in Health Care: A Report from the National Academy of Medicine. JAMA. 2020;323:509-10.
- [CrossRef] [Google Scholar]