Translate this page into:
Artificial Intelligence in Echocardiography: Where Do We Stand?
*Corresponding author: Devishree Das, Department of Anaesthesiology and Critical Care, All India Institute of Medical Sciences, Bhubaneswar, Odisha, India. devishreedas111@gmail.com
-
Received: ,
Accepted: ,
How to cite this article: Das D, Sahoo S. Artificial Intelligence in Echocardiography: Where Do We Stand? J Card Crit Care TSS. 2025;9:84-91. doi: 10.25259/JCCC_59_2024
Abstract
Artificial intelligence (AI) has been expanding exponentially in the field of health care. AI not only simplifies disease interpretation but also improves the efficiency of patient management. The novel machine learning algorithms, and deep learning models are expanding the boundaries in the arena of echocardiography. The automated assessment of the biventricular function, atrio-ventricular coupling, Integrating novel approaches like speckle tracking may aid in the identification, classification, diagnosis, and prognostication of cardiovascular abnormalities. Moreover, AI integration reduces the time of interpretation, inter as well as intra-observer variability and provides a rapid, non-invasive and accurate result. AI stands at the pinnacle of echocardiography. Therefore, the index article aims to review the existing and upcoming AI integration modalities in echocardiography with regard to the technique, advantages, limitations, and its clinical application.
Keywords
Artificial intelligence
Cardiovascular abnormalities
Deep learning
Echocardiography
Machine learning
INTRODUCTION
Artificial intelligence (AI) has ushered in a new era of echocardiography. Integration of AI datasets aids in unmatched efficiency and reproducibility in the arena of echocardiography, making an invaluable modality for meeting increased demand. The contribution of novel machine learning (ML) models, and deep learning (DL) algorithms are transformative, as these can improve and generate new prognostication modalities for various cardiac as well as cardiovascular abnormalities.
Echocardiography provides non-invasive, accurate, and immediate characterization of cardiac anatomy, evaluation of biventricular function, atrioventricular coupling, valvular function, delineation of the pulmonary vasculature, and congenital abnormalities.[1] Its availability all around, minimal price, and better safety profile made it a necessary tool for all clinicians, including cardiologists and perioperative cardiac anesthesiologists.[2] Transesophageal echocardiography not only confirms the diagnosis but also delineates the anatomy and guides surgical repair. AI integration further minimizes the time of acquisition, and automated border tracking enables accurate assessment of cardiac function in a time constraint perioperative period and, therefore, considered as a modern stethoscope in the armamentarium of cardiac anesthesiologists. AI integration can also provide additional information which the human eye fails to detect.
In echocardiography, the interpretation of cardiac function highly depends on the subjective knowledge and level of experience of the interpreter as compared to other imaging techniques such as nuclear imaging, computed tomography, and magnetic resonance imaging. This limitation can be overcome by AI technologies that offer produce automated, and more consistent interpretations of echocardiography.[3,4] Furthermore, AI has been used in educating and training novice doctors for image acquisition and interpretation.[5,6]
AI: THE INNOVATION
AI defines the application of machines to simulate the human brain and carry out multiple activities with limited participation or supervision.[7] ML is a subfield of AI which allows analysis of wide datasets through computing and various statistical algorithms. Furthermore, ML models can provide predictions and prognostication on the basis of unseen datasets.[8]
ML technique is categorized into three major groups, unsupervised, supervised, and reinforcement learning. Unsupervised learning mainly uses unlabeled datasets and focuses on devising new patterns and linkage among variables, whereas supervised learning “taught” machine to group the data by providing labeled data. Furthermore, the reinforcement learning model uses algorithms that are obtained through a trial and error method with only given dataset to optimize the result [Figure 1].
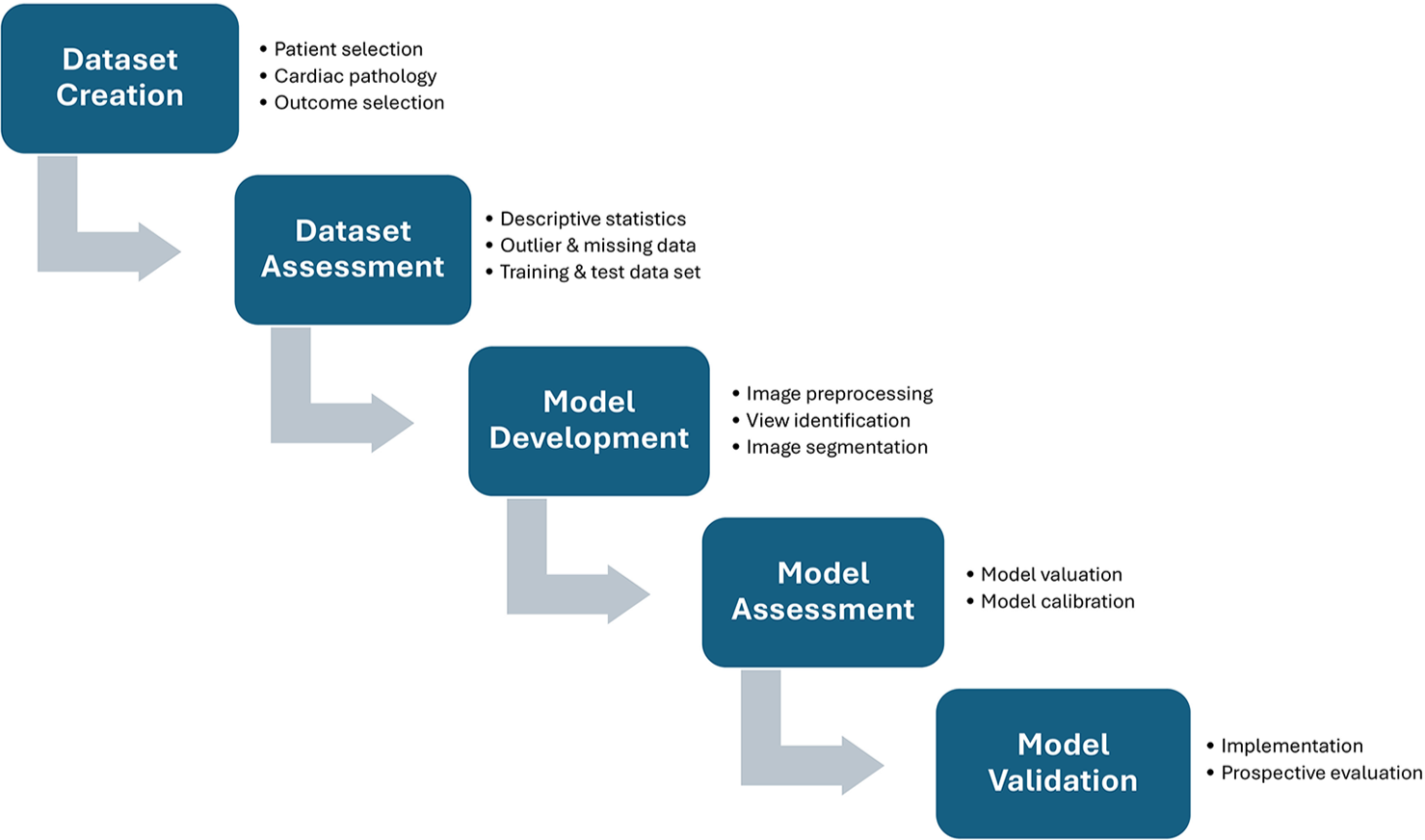
- Deep learning workflow in automated image analysis.
DL algorithm is a subcategory of ML model which consists of networks of nodes which simulate the human brain and neural networking, therefore, called artificial neural networks. Commonly, DL model constitutes convolutional neural networks (CNN) and recurrent neural networks. CNN can able to process two-dimensional (2D) image on the basis of multi-layered datasets, whereas recurrent neural networks are utilized for sequential datasets which, therefore, can be used for the interpretation of language and speech recognition.
AI INTEGRATED ECHOCARDIOGRAPHY: THE TIME IS NOW
Echocardiographic views acquisition and interpretation
AI algorithms in integration with commercially accessible software play a key role in acquisition and interpretation of echocardiographic views. These AI integrated models provide clear instructions for image acquisition, with proper probe manipulation, recognizing and warning about the image quality, thus facilitating training as well as self-improvement [Table 1]. In 2021, Narang et al.[5] expressed the use of DL model in training nurses for the acquisition of echocardiograms and these nurses have no prior exposure to echocardiography. Same year, Schneider et al.[6] trained 1st year medical students about the acquisition of diagnostic echocardiographic views using ML model; furthermore, this ML algorithm calculates left ventricle ejection fraction (LVEF) from the acquired datasets. Madani et al.[9] applied CNN for developing AI algorithm to classify 15 classical views on the basis of 267 labeled trials with a clinical variation. Their model can classify the images with 97.8% accuracy. More importantly, the interpretation is rapid with an average of 21 milliseconds per image. Zhang et al.[10] also trained and validated CNN models for multi-tasking in echocardiography that includes classification of 23 standard views.
S. No. | Authors | Type of AI algorithm | Description |
---|---|---|---|
1. | Narang et al.[5] | DL algorithm | Guidance on image acquisition |
2. | Schneider et al.[6] | ML algorithm | Guidance for acquiring diagnostic echocardiography images |
3. | Madani et al.[9] | CNN model | Classification of 15 standard views |
4. | Zhang et al.[10] | CNN models | Classification of 23 standard views and segmentation |
5. | Leclerc et al.[11] | CNN DL model | Segmentation and analysis of apical four and two chamber views to measure LV EDV, ESV, and EF |
6. | Ouyang et al.[12] | CNN model | Prediction of EF |
7. | Salte et al.[13] | DL model | Automated segmentation and measurement of GLS |
8. | Salem Omar et al.[14] | AI model | Prediction of increased LV filling pressure |
9. | Pandey et al.[15] | ML model | Prediction of elevated LV filling pressure |
10. | Zhu et al.[16] | AI algorithm | Assessment of RV function using 3D echocardiography |
11. | Shad et al.[17] | Video-based DL model | Development of RV failure |
12. | Moghaddasi and Nourian[18] | ML model | Assessment of mitral regurgitation |
13. | Prihadi et al.[19] | 3D AI model | Measurement of aortic annulus and root dimensions |
14. | Queiros et al.[20] | AI algorithm | Aortic valve assessment for TAVR |
15. | Omar et al.[21] | DL based algorithm | Strain analysis for stress echocardiograms |
16. | Upton et al.[22] | CNN model | Prognostication of coronary artery disease on stress echocardiograms |
17. | Ghorbani et al.[23] | CNN model | Diagnosing pacemaker leads, left atrial enlargement, and LV hypertrophy |
18. | Kusunose et al.[24] | CNN model | Detection of regional wall motion abnormality |
19. | Strzelecki et al.[25] | AI-derived algorithm | Automatic identification of intracardiac tumor and thrombi |
20. | Sun et al.[26] | Computer-aided diagnostic algorithm | Left atrial and left atrial appendage thrombi |
21. | Samad et al.[27] | Non-linear ML model | Prediction of survival from tricuspid regurgitation velocity than EF |
AI: Artificial intelligence, DL: Deep learning, ML: Machine learning, CNN: Convolutional neural networks, LV: Left ventricle, RV: Right ventricle, ESV: End-systolic volume, EDV: End-diastolic volume, EF: Ejection fraction, GLS: Global longitudinal strain, TAVR: Transcatheter aortic valve replacement, 3D: Three-dimensional
ECHOCARDIOGRAPHIC IMAGE ANALYSIS AND INTERPRETATION
Left ventricular systolic function (LVSF)
LVSF is one of the primary echocardiographic derivative, which has significant prognostic value. Systolic and diastolic function is the two horizon of heart failure, the determination of which comprises high inter- as well as intra-observer variability and poor reproducibility. The most commonly used modified Simpson’s biplane method for calculation of LVEF required manual tracing of end-systolic and enddiastolic border of the LV in the orthogonal views such as four-chamber and two-chamber views. It is challenging at times as it depends on good quality images, time consuming for manual tracing and has interobserver variability.
Leclerc et al.[11] trained an encoder decoder-based CNN and DL model for segmentation and analysis of 500 echocardiography with four- and two-chamber views and able to measure end-systolic, end-diastolic volumes, and LVEF. Its reproducibility was superior with regard to inter-observer variability than conventional methods. Similarly, Ouyang et al.[12] developed another CNN model with 10030 apical four chamber echocardiographic loops, which can predict LVEF with a mean absolute error of 4.1% and much faster rate, that is, 1.6 s per cardiac cycle [Figure 2 and 3].
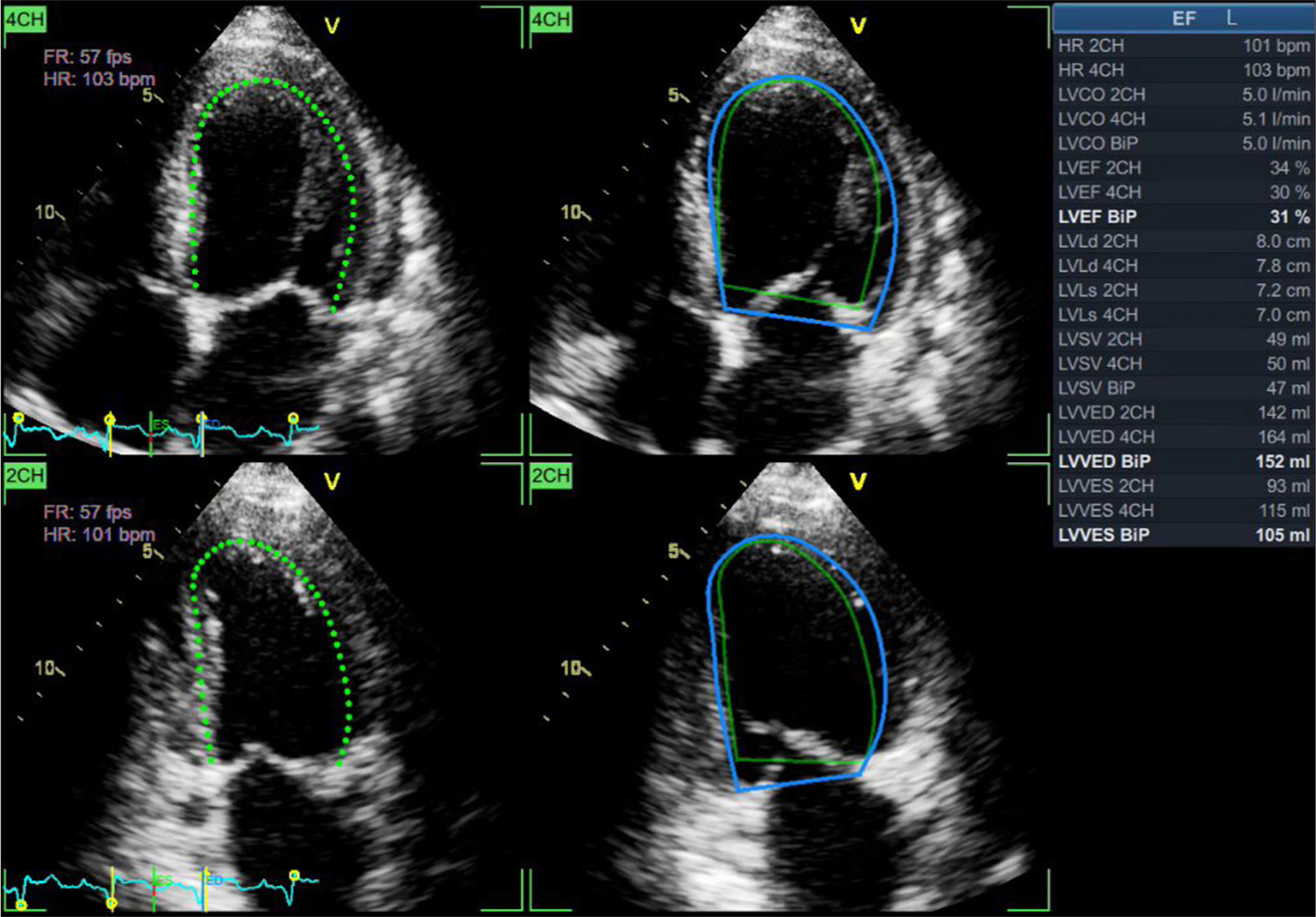
- Artificial intelligence integrated calculation of ejection fraction by automated border tracking. Green dots represents the endo-myocardial border whereas green and blue solid lines denote end-systolic and end-diastolic border respectively.
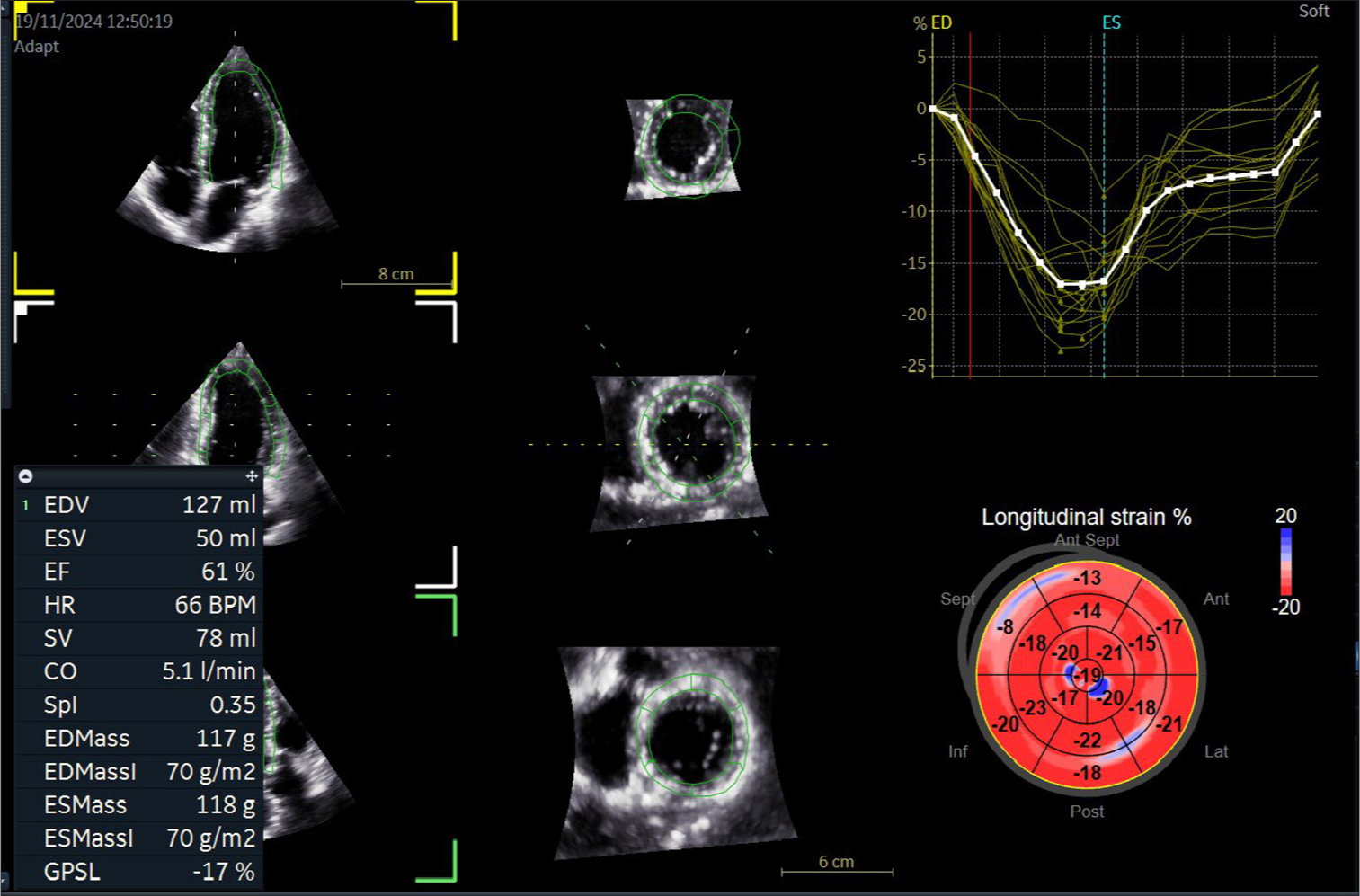
- Artificial intelligence integrated three-dimensional quantification of left ventricular function.
LV Strain
Salte et al.[13] developed a DL-based algorithm to estimate global longitudinal strain (GLS) using traditional 2D echocardiography. The DL model effectively accomplished automatic segmentation and estimations of GLS across a wide range of cardiac abnormalities, with little variation of 1.8% between the methods [Figure 4]. The evaluation was rapid and takes hardly 15 s time per assessment as compared to 5–10 min by traditional technique to determine GLS.
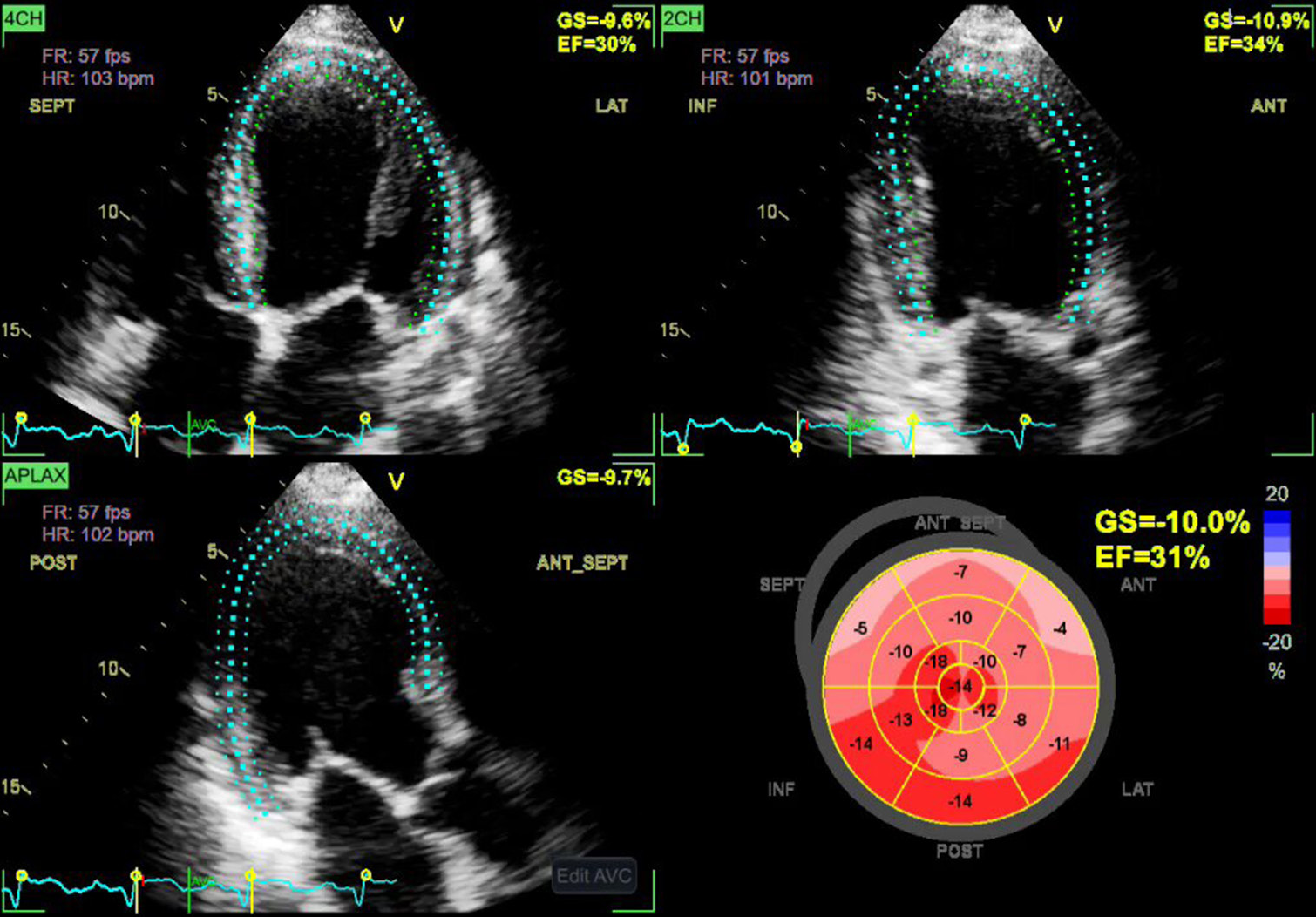
- Artificial intelligence integrated measurements of global longitudinal strain by automatic speckle tracking. Blue dots indicate “speckles”.
LV Diastolic function
Assessment of diastolic function is extremely difficult, with multiple echocardiographic parameters, numerous complex flow charts, and critical appraisal with guidelines. The novice AI technology enables easy diagnosis and interpretation of diastolic dysfunction. Salem et al.[14] used speckle tracking echocardiographic measurements to create an AI model that can precisely predict increased left atrial pressure, the key variable of diastolic dysfunction. Pandey et al.[15] emphasized ML-based algorithm for identifying individuals with high left atrial pressure in comparison to the American Society of Echocardiography 2016 diastolic guidelines grading system.
Right ventricle (RV) function
Evaluation of RV function is laborious and is affected by congenital malformation, left ventricular failure, valvular abnormalities, pulmonary arterial hypertension, and coronary artery disease (CAD). Moreover, precise evaluation of RV dysfunction may be tough due to its crescent shape, poor echocardiographic delineation of the RV, and discrepancy in RV functional analysis. However, with the advancement of AI technology, RV function can be assessed accurately and in a faster time. Zhu et al.[16] formulate an AI-based 3D echocardiographic algorithm to evaluate RV function accurately. The AI model showed excellent diagnostic accuracy being cut off ejection fraction of 43% with sensitivity and specificity of 94% and 67%, respectively, in comparison to cardiac magnetic resonance 0imaging [Figure 5]. Following LV assist device implantation, RV dysfunction is common but difficult to predict on the basis of existing echocardiographic parameters. However, Shad et al.[17] used video-based DL to forecast the development of RV failure following device implantation utilizing 2D echocardiographic dataset.
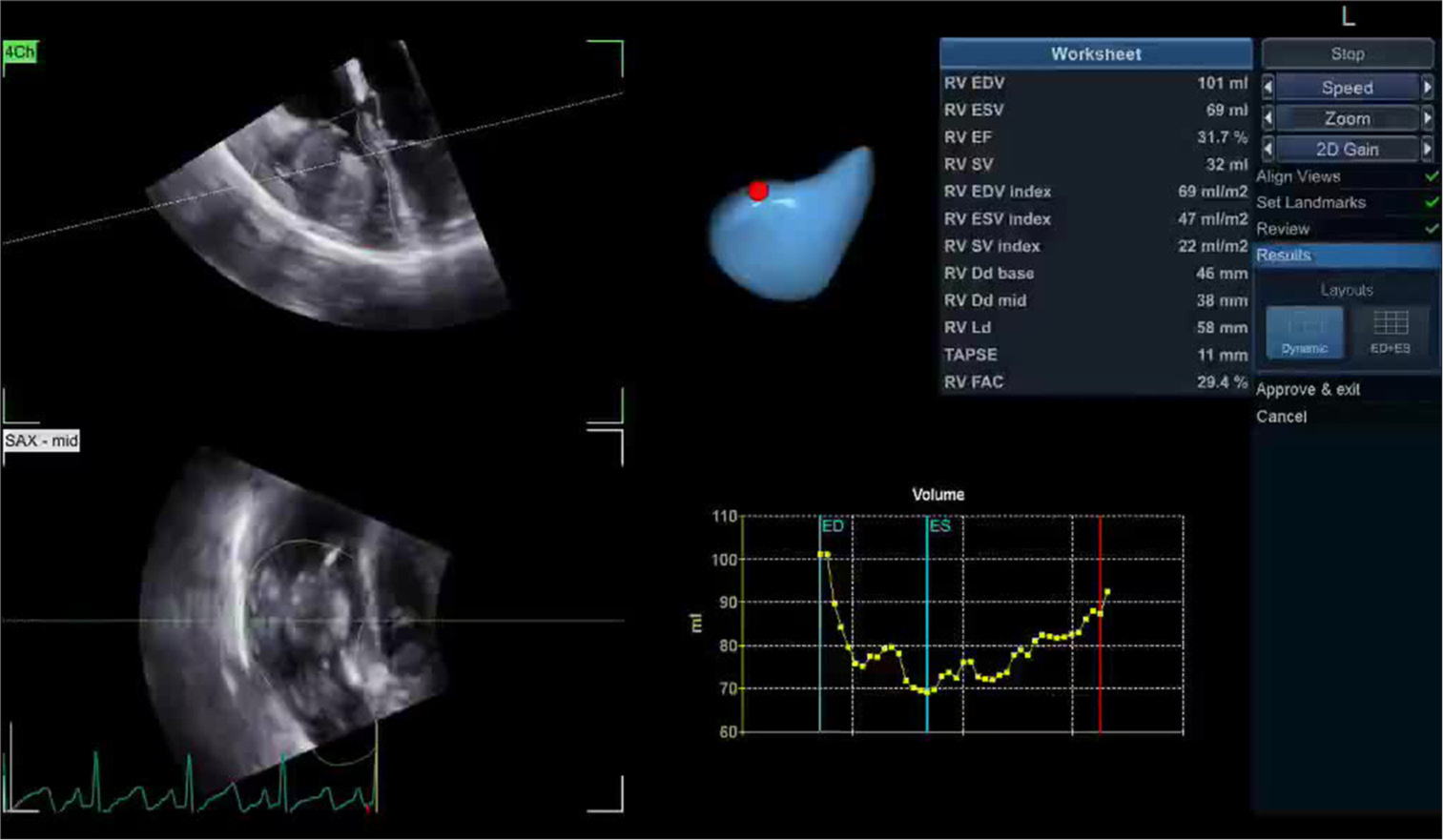
- Artificial intelligence integrated three-dimensional quantification of right ventricular function.
Valvular function
Echocardiographic evaluation of valvular morphology and function is a tedious process which requires proper imaging and precise measurements for the feasibility of repair. Moghaddasi and Nourian[18] used ML model for the assessment of mitral regurgitation on the basis of image processing and micro-patterns of 2D echocardiography [Figure 6[. Prihadi et al.[19] innovate a AI based 3D transesophageal echocardiographic technique for precise measurement of aortic root and annular dimensions. Similar findings were also demonstrated by Queiros et al.[20] but using a different AI model for assessment of aortic valve evaluation in transcatheter aortic valve replacement.
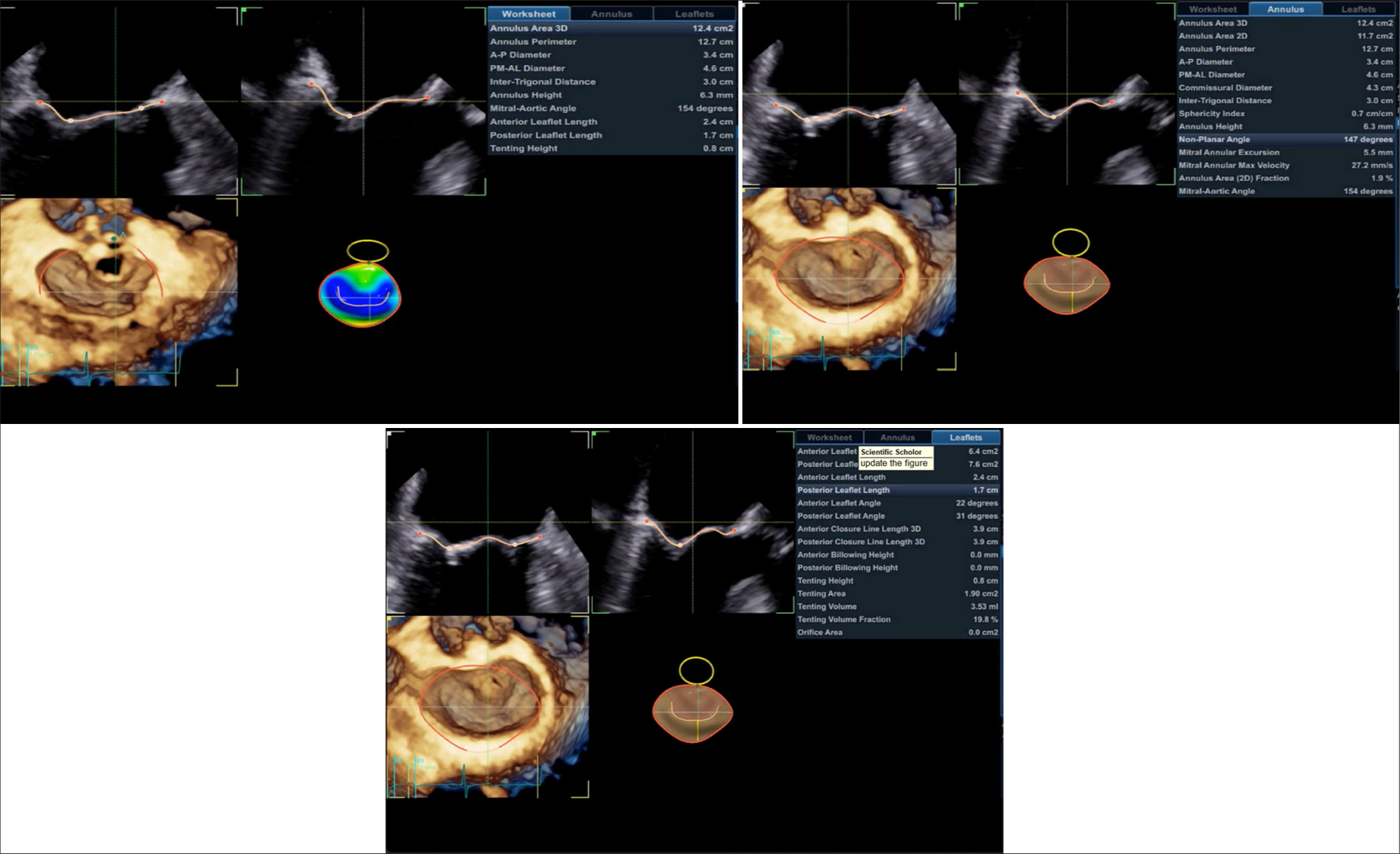
- Artificial intelligence integrated three-dimensional quantification of mitral valve.
Stress echocardiography
Stress echocardiography is a convenient technique for the detection of CAD, but requires a substantial learning with persistent inter-observer variability. Omar et al.[21] confirmed the effectiveness of DL-based algorithm, which can be used for analyzing strain of stress echocardiographs. Upton et al.[22] did a multi-centric, multi-vendor trial using a CNN model that can recognize angiographically confirmed CAD on stress echocardiograms.
Other utilities
Zhang et al.[10] used a CNN-based model to develop a fully automated echocardiographic modality, including image recognition and segmentation to diagnose hypertrophic obstructive cardiomyopathy, amyloidosis, and pulmonary hypertension. Ghorbani et al.[23] invented a novice CNN algorithm to identify pacemaker leads, left atrial enlargement, and LV hypertrophy. Omar et al.[21] customized a CNN technique for the automatic assessment of regional wall motion abnormality by analyzing strain during dobutamine stress echocardiograms to diagnose CAD. Similarly, Kusunose et al.[24] developed CNN algorithm for distinguishing regional wall motion abnormality. Strzelecki et al.[25] validated an AI-derived model for automatic recognition of various intracardiac tumor and mass using 2D echocardiography. This AI model demonstrates better accuracies, sensitivities, and specificities than conventional echocardiography. Sun et al.[26] developed a computer-aided diagnostic model from transesophageal echocardiographic images to detect left atrial and left atrial appendage thrombi. AI models can be integrated into hemodynamic measurements in less time. ML models trained to calculate systemic vascular resistance (SVR), pulmonary vascular resistance (PVR), and cardiac output (CO). Furthermore, AI-enhanced Doppler echocardiography can refine the TR jet, can integrate cardiac MRI, and catheterization data for accurate estimation of PVR. Similarly, AI-driven algorithms can predict SVR in real time, enabling vasopressor and fluid titration.
Disease prognostication
Samad et al.[27] developed a non-linear ML algorithm for the prediction of survival by utilizing clinical parameters and echocardiographic datasets. They have concluded that the tricuspid regurgitation velocity was more reliable echocardiographic parameter for survival than LVEF. Furthermore, Omar et al.[21] utilized unsupervised cluster analysis techniques for the evaluation of diastolic function and found two phenotypic categories of diastolic failure. Zhang et al.[10] used AI-derived echocardiographic paraments for the assessment of GLS in individuals treated with cardiotoxic chemotherapeutic agents and even prognostication of the patients.
AI-BASED TRAINING
AI has made immense development in the training for the acquisition, interpretation, and diagnosis of cardiovascular abnormalities by echocardiography. The major issue is to train how to operate the machine as well as the probe. Arbeille et al.[28] first revealed the efficient control of robots with a teleoperated motorized echocardiography probe by trained echocardiographers. Later on, Narang et al.[5] and Schneider et al.[6] used AI-based technology to train nurses and 1st year medical students, respectively.
OVERCOMING CHALLENGES: THE FUTURE PROSPECTS
Despite of huge development of AI in echocardiography, there are certain challenges for its globalization. Firstly, legal and ethical issues incurred with AI integration on echocardiography are the major hurdle; therefore, extensive validation study should be carried out before seeking approval of regulatory bodies.[29]
Furthermore, the internal network of DL algorithms is difficult to understand, therefore, often considered as a “black box” that makes hesitancy in adoption by clinicians. Although challenging and time-consuming, extensive training with larger training data sets with regard to acquisition, labeling, and interpretation can overcome the hesitancy hurdle.[30]
The most important limitation is the requirement of huge data banks constituting high quality training datasets to train the algorithm. If the algorithm is trained with sub-optimal real world imaging, it will give impaired view recognition and interpretation, which can hamper the quality of results. The solution is to continue continuous training of models with real world datasets to ascertain improved effectuality and safety.[31-33]
Another limitation is paucity of clinical trials on AI. Evidence of robust clinical outcomes should be required before the integration of AI into echocardiographic practice. Moreover, more clinical trials are required to validate AI in multiple demographic locations and various vendor-dependent setups. AI-enabled systems are costlier to set up; however, carrying out repetitive, simple tasks more accurately and substantially faster without medical errors make it cost effective.
AI models trained primarily on normal sinus rhythm patients and may not generalize well to patients with arrhythmias unless explicitly trained with diverse dataset. However, AI can analyze thousands of beats quickly and provide trend analysis rather than relying on single cycle measurement. Future AI models may integrate real-time electrocardiogram and respiratory monitoring to adjust these variations dynamically.
AI is continuously evolving and therefore, a multidisciplinary approach with engineers, computer scientists, and echocardiographers is inevitable for the fruitful integration of AI in echocardiography. This novice innovation expanded its boundaries and paved the pathway for huge clinical studies as well as multicentric trials.
CONCLUSION
Transforming potential of AI has led its integration with echocardiography. Starting from training echocardiographers, AI integration culminate improvement in image acquisition, recognition, and interpretation of echocardiographic views. The major perk of AI integration is incomparable effectiveness and reproducibility. Despite of considerable impediments, the future of AI in echocardiography cannot be challenged and has the potential to revolutionize modern echocardiography.
Ethical approval
Institutional Review Board approval is not required.
Declaration of patient consent
Patient’s consent not required as there are no patients in this study.
Conflicts of interest
There are no conflicts of interest.
Use of artificial intelligence (AI)-assisted technology for manuscript preparation
The authors confirm that there was no use of artificial intelligence (AI)-assisted technology for assisting in the writing or editing of the manuscript and no images were manipulated using AI.
Financial support and sponsorship: Nil.
References
- International Evidence-Based Recommendations for Focused Cardiac Ultrasound. J Am Soc Echocardiogr. 2014;27:683.e1-33.
- [CrossRef] [PubMed] [Google Scholar]
- Handheld Point-of-Care Ultrasound Probes: The New Generation of POCUS. J Cardiothorac Vasc Anesth. 2020;34:3139-45.
- [CrossRef] [PubMed] [Google Scholar]
- Machine Learning for Prediction of All-cause Mortality in Patients with Suspected Coronary Artery Disease: A 5-year Multicentre Prospective Registry Analysis. Eur Heart J. 2017;38:500-7.
- [CrossRef] [PubMed] [Google Scholar]
- Current Challenges and Recent Updates in Artificial Intelligence and Echocardiography. Curr Cardiovasc Imaging Reports. 2020;13:5.
- [CrossRef] [Google Scholar]
- Utility of a Deep-Learning Algorithm to Guide Novices to Acquire Echocardiograms for Limited Diagnostic Use. JAMA Cardiol. 2021;6:624-32.
- [CrossRef] [PubMed] [Google Scholar]
- A Machine Learning Algorithm Supports Ultrasound-naïve Novices in the Acquisition of Diagnostic Echocardiography Loops and Provides Accurate Estimation of LVEF. Int J Cardiovasc Imaging. 2021;37:577-86.
- [CrossRef] [PubMed] [Google Scholar]
- Cardiovascular Imaging and Intervention Through the Lens of Artificial Intelligence. Interv Cardiol. 2021;16:e31.
- [CrossRef] [PubMed] [Google Scholar]
- Artificial Intelligence in Cardiology. J Am Coll Cardiol. 2018;71:2668-79.
- [CrossRef] [PubMed] [Google Scholar]
- Fast and Accurate View Classification of Echocardiograms Using Deep Learning. NPJ Digit Med. 2018;1:6.
- [CrossRef] [PubMed] [Google Scholar]
- Fully Automated Echocardiogram Interpretation in Clinical Practice. Circulation. 2018;138:1623-35.
- [CrossRef] [PubMed] [Google Scholar]
- Deep Learning for Segmentation Using an Open Large-Scale Dataset in 2D Echocardiography. IEEE Trans Med Imaging. 2019;38:2198-210.
- [CrossRef] [PubMed] [Google Scholar]
- Video-based AI for Beat-to-beat Assessment of Cardiac Function. Nature. 2020;580:252-6.
- [CrossRef] [PubMed] [Google Scholar]
- Artificial Intelligence for Automatic Measurement of Left Ventricular Strain in Echocardiography. JACC Cardiovasc Imaging. 2021;14:1918-28.
- [CrossRef] [PubMed] [Google Scholar]
- Artificial Intelligence-Based Assessment of Left Ventricular Filling Pressures From 2-Dimensional Cardiac Ultrasound Images. JACC Cardiovasc Imaging. 2018;11:509-10.
- [CrossRef] [PubMed] [Google Scholar]
- Deep-Learning Models for the Echocardiographic Assessment of Diastolic Dysfunction. JACC Cardiovasc Imaging. 2021;14:1887-900.
- [CrossRef] [PubMed] [Google Scholar]
- Quantitative Assessment of Right Ventricular Size and Function with Multiple Parameters from Artificial Intelligence-based Three-dimensional Echocardiography: A Comparative Study with Cardiac Magnetic Resonance. Echocardiography. 2022;39:223-32.
- [CrossRef] [PubMed] [Google Scholar]
- Predicting Post-operative Right Ventricular Failure Using Video-based Deep Learning. Nat Commun. 2021;12:5192.
- [CrossRef] [PubMed] [Google Scholar]
- Automatic Assessment of Mitral Regurgitation Severity Based on Extensive Textural Features on 2D Echocardiography Videos. Comput Biol Med. 2016;73:47-55.
- [CrossRef] [PubMed] [Google Scholar]
- Feasibility, Accuracy, and Reproducibility of Aortic Annular and Root Sizing for Transcatheter Aortic Valve Replacement Using Novel Automated Three-Dimensional Echocardiographic Software: Comparison with Multi-Detector Row Computed Tomography. J Am Soc Echocardiogr. 2018;31:505-14.e3.
- [CrossRef] [PubMed] [Google Scholar]
- Fully Automatic 3-D-TEE Segmentation for the Planning of Transcatheter Aortic Valve Implantation. IEEE Trans Biomed Eng. 2017;64:1711-20.
- [CrossRef] [PubMed] [Google Scholar]
- 'Quantification of Cardiac Bull's-eye Map based on Principal Strain Analysis for Myocardial Wall Motion Assessment in Stress Echocardiography In: 2018 IEEE 15th International Symposium on Biomedical Imaging (ISBI 2018), Washington, DC, USA. 2018. p. :1195-1198.
- [CrossRef] [Google Scholar]
- Automated Echocardiographic Detection of Severe Coronary Artery Disease Using Artificial Intelligence. JACC Cardiovasc Imaging. 2022;15:715-27.
- [CrossRef] [PubMed] [Google Scholar]
- Deep Learning Interpretation of Echocardiograms. NPJ Digit Med. 2020;3:10.
- [CrossRef] [PubMed] [Google Scholar]
- A Deep Learning Approach for Assessment of Regional Wall Motion Abnormality from Echocardiographic Images. JACC Cardiovasc Imaging. 2020;13:374-81.
- [CrossRef] [PubMed] [Google Scholar]
- Classification and Segmentation of Intracardiac Masses in Cardiac Tumor Echocardiograms. Comput Med Imaging Graph. 2006;30:95-107.
- [CrossRef] [PubMed] [Google Scholar]
- A Computer-aided Diagnostic Algorithm Improves the Accuracy of Transesophageal Echocardiography for Left Atrial Thrombi: A Single-center Prospective Study. J Ultrasound Med. 2014;33:83-91.
- [CrossRef] [PubMed] [Google Scholar]
- Predicting Survival From Large Echocardiography and Electronic Health Record Datasets: Optimization With Machine Learning. JACC Cardiovasc Imaging. 2019;12:681-9.
- [CrossRef] [PubMed] [Google Scholar]
- Teles-operated Echocardiography Using a Robotic Arm and An Internet Connection. Ultrasound Med Biol. 2014;40:2521-9.
- [CrossRef] [PubMed] [Google Scholar]
- Current Role and Future Perspectives of Artificial Intelligence in Echocardiography. World J Cardiol. 2023;15:284-92.
- [CrossRef] [PubMed] [Google Scholar]
- The Role of Artificial Intelligence in Echocardiography. J Imaging. 2023;9:50.
- [CrossRef] [PubMed] [Google Scholar]
- Artificial Intelligence-based Classification of Echocardiographic Views. Eur Heart J Digit Health. 2024;5:260-9.
- [CrossRef] [PubMed] [Google Scholar]
- Artificial Intelligence in Cardiac Critical Care: Current Insights and Future Prospects. J Card Crit Care TSS. 2024;8:189-94.
- [CrossRef] [Google Scholar]
- Artificial Intelligence in POCUS: The Vanguard of Technology in COVID-19 Pandemic. J Card Crit Care TSS. 2020;4(2):116-120.
- [CrossRef] [Google Scholar]